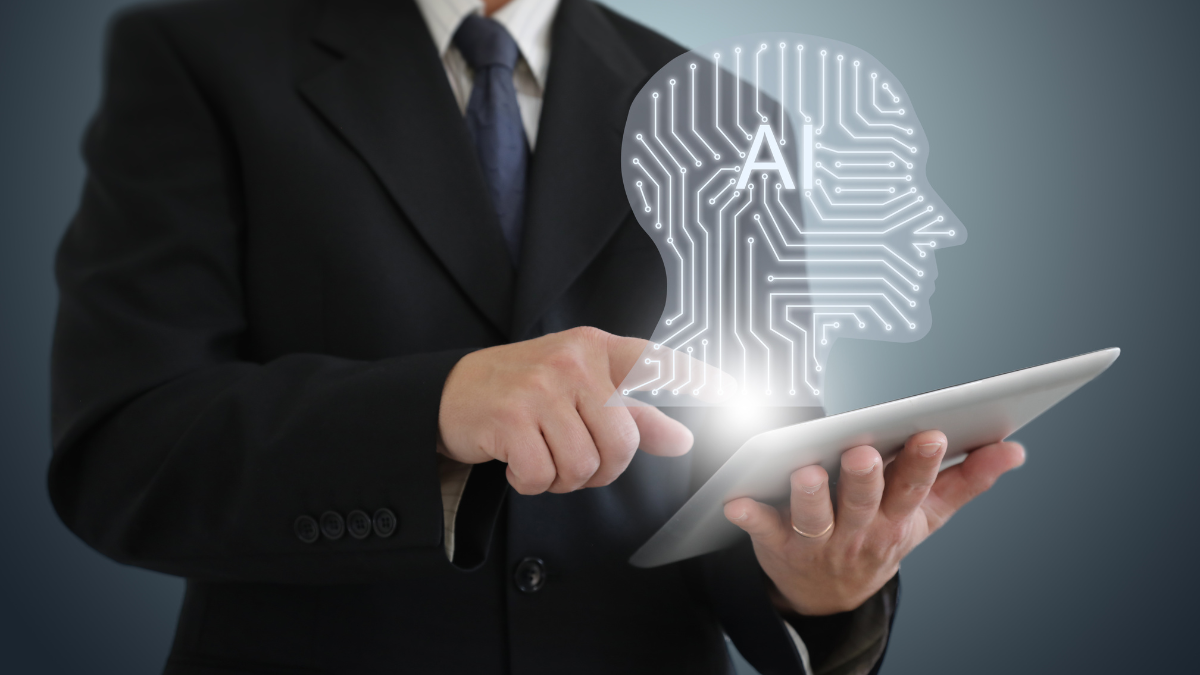
The mortgage servicing industry faces significant challenges in managing cash flows efficiently. In real terms, the crux of the problem isn’t so much inefficiencies as it is transparency into the extent of the problem — in investor accounting, what you don’t know can hurt you. Lenders, investors, and borrowers are all impacted by this lack of transparency as it often results in delayed transactions, increased financial risks, and compliance challenges.
The velocity and complexity of mortgage transactions make it difficult to track and resolve discrepancies, often resulting in substantial cash flow variances. For instance, investor accounting represents about 40% of GSE scorecard metrics but only about 2% of a servicer’s cost structure. That is a significant mismatch.
Independent Mortgage Banks (IMBs) dominate the origination sector, accounting for over 80% of all new mortgages. These loans are frequently sold to entities like Fannie Mae and managed by large companies. The IMB or bank will aggregate a portfolio for several months and then sell their mortgage servicing rights to an MSR REIT, and the cash flows exchange hands again. To further complicate things, these loans are sold to the GSEs and GNMA with various types of remittances which dictate how cash flows are treated for scheduled or actual principal and interest handling.
All this clouds the visibility of actual data by adding layers and layers of subservicing functions. The excess servicing strip can be further carved up between the servicing fee, guarantee fee, and excess or interest-only. And we’re not even done.
Now, overlay borrower behavior—some loans are delinquent, some are prepaid, some have curtailments, etc. — and these all need to be normalized based on the remittance type so the investor is assured of its cash flows and the GSEs’ custodial accounts are properly funded.
So how can the mortgage industry cut through this complexity and get a clear view of things? Fortunately, there’s a simple answer, a new tool that makes it possible to quickly process huge swaths of data and see a sharp picture — it’s called Narrow AI.
The transparency dilemma
Think of cruising in a boat in placid waters. It’s a sunny day, but you look ahead and see an iceberg floating on the horizon. It’s an unexpectedly beautiful sight to see… but a knowledgeable captain steers wide because she knows only 10% of the mass can be seen, while 90% — the riskiest part — is below the waterline. Investor accounting is no different.
Our segment of the mortgage industry is unique in that it requires very high precision due to the complexity of the accounting requirements. We must ensure the cash flows we manage are accurate with absolute certainty. Even a small discrepancy can have drastic results.
Over the past five years, we’ve observed an average of 1.5% of the loans in a portfolio having an exception each month. This includes reporting exceptions, loan attribute exceptions, or cash flow variances between the investor and the servicer. These exceptions have ranged from as little as 0.5% to over 6% on any given month, driven mostly by human error when touching a loan during transitions, transactions, and other terminal events.
While a 1.5% exception rate might not seem like a large number in isolation, when applied to several million loans each month, it equates to 45,000 items to research. And remember, that’s just an average. This, in turn, represents almost three-quarters of a billion dollars in cash flow variances to research over the course of a year.
That is very real money, yet very few organizations can even quantify it because they lack transparency into the problem. They’re looking in Excel spreadsheets, often using freeform notes to describe their research with little additional detail on the cause and effect of why they labeled it as such. When they run out of time, they succumb to the temptation to copy and paste — or worse, to plug.
Introducing Narrow AI
When people think of AI, they immediately think of ChatGPT. ChatGPT is a multimodal generative AI tool that does many things well but is expert in very little. When you benchmark these generative AI tools against specific disciplines such as business, science, and the arts, they still trail human capabilities by a gap of 30% or more. While they’re closing this gap quickly, there is still a significant amount of time expected before AI of this type can be trusted with high-precision tasks like the ones we face.
Narrow AI is a category of AI systems that is purpose-built for a specific task — mathematics, decision analysis, etc. Think of them as specialists, while ChatGPT is a generalist. Narrow AI is created through thorough research and testing of leading open-source, special-purpose ML algorithms designed to excel at your use case. But they must be carefully calibrated and trained with your data.
Machine learning of this nature requires huge amounts of data and extreme care to ensure that data is of the highest possible quality. Using bad data in a machine learning training scenario is essentially the same as teaching a new employee the wrong way to complete a task.
Our world is one of exacting precision. A good profile for a person adept at Investor Accounting is someone with a strong predisposition to problem-solving, excellent math skills, a high propensity to learn, and someone who innately cares about every tiny detail.
The machines we want to do our work are no different — we want to hang out with the math nerd machine, not the well-rounded popular one.
AI in Action: Success Stories
For over a decade, we’ve built systems from an analytical use case perspective. The challenge is how to maintain high-quality, robust data over long periods so we can continuously harness the power of that underlying data. At PMSI, we’ve achieved that at great expense in time, effort, and funding to create Narrow AI capable of greatly reducing the time it takes to comb through all of this data. Our researchers — assisted by our expert Narrow AI algorithms — are able to perform more checks and balances than a standard operation.
Therefore, we catch more.
Some examples of our achievements using these methods include:
- Mitigating $6M in data discrepancies and cash variances in portfolio transfers that would have otherwise been accepted by the client because we could identify and quantify the 1/10 of 1% that was deficient.
- Returning over $38M in unnecessary P&I advances for one client on one GSE portfolio.
- Elevating clients from worst to first in their GSE scorecards, achieving sustained FNMA Star rankings and FHLMC Sharp rankings.
- Resolving 24,000 pool reporting exceptions in 3.5 hours to prevent GNMA penalties.
- Researching over $60 million in cash variances each month, with our algorithms automating 75% to 85% of the research.
The future of AI in mortgage servicing
There are no two ways around it — integrating AI into your workflow will make your mortgage servicing operation more efficient. There are areas today where AI equals or exceeds human capabilities — image recognition and text comprehension, to name a few. You should be focusing on integrating these capabilities into your workflow today, such as enlisting AI for document classification during loan transfers and loan boarding.
Within call centers, chat lines, and regulatory guidelines, AI can be utilized for data mapping and document parsing—you only have to use your imagination to find places where an AI can speed up processes by offloading work from your staff.
For Narrow AI applications like those detailed above, it’s honestly too late to start building your own solutions. By the time you have amassed the necessary data for training and gone about ensuring that it meets the strictest quality standards, the innovators in the space will be on to next-generation models that will far outpace anything you’re starting now. But it’s certainly time to reach out to these innovators and explore how their technologies could be used to help your business get ahead.
Simply put, AI is transforming the mortgage servicing industry as rapidly and radically as it is transforming so much else around us. It’s time to get on board and find ways to implement AI in your own workflows.
Daniel Thompson is the CEO of PMSI.
This column does not necessarily reflect the opinion of HousingWire’s editorial department and its owners.
To contact the editor responsible for this piece: [email protected].
First Time Home Buyer FAQs - Via HousingWire.com